Introduction:
It is a set of techniques used by researchers to separate and examine sequence data from individual cells. Single cell sequencing techniques were recognized as the Nature Method of the Year in 2013 and have helped scientists understand more than ever before about how cells function. With the help of a technology called single-cell sequencing, it is possible to sequence the DNA, RNA, or epigenetic alterations of a single cell, obtaining high-resolution data on the genetic and molecular properties of a single cell within highly organised biological systems (tissues/organs/organisms).
The study of genomic and transcriptome variability at the single cell level has provided novel insights into a variety of fields of study, including cancer research, cell growth and function, and immunology.
Discovery:
Several key advances in genomics and sequencing technologies have contributed to the discovery of single-cell sequencing. While early efforts to sequence individual genes from single cells were made in the 1990s using PCR-based methods, genome-wide analysis of individual cells was not possible until the development of next-generation sequencing (NGS) technologies in the 2000s.
The Broad Institute and Harvard University published the first demonstration of genome-wide single-cell sequencing in 2009, using microfluidic equipment to isolate and analyse the transcriptomes of single human embryonic stem cells. This study showed that single-cell sequencing has the ability to identify unusual cell types and analyse gene expression heterogeneity within complex tissues. Several other single-cell sequencing technologies were developed in the years since, including scRNA-seq, scDNA-seq, and scATAC-seq.
Principle:
Single-cell sequencing is based on the generation of genomic information from individual cells in order to analyse their genetic and molecular features. Researchers are able to examine the heterogeneity of complex biological systems at a higher level, reveal unusual cell populations, and better understand dynamic cellular processes using this approach. Single-cell sequencing workflow includes numerous essential phases, such as cell separation, cell lysis, amplification, library preparation, sequencing, and data analysis, which might vary depending on the type of single-cell sequencing done. Overall, single-cell sequencing is a potent technique that has transformed genomics and has numerous applications in biology and medicine.
Types:
There are several types of single-cell sequencing, each of which focuses on a different type of genomic information. Some of the most common types of single-cell sequencing include:
Single-cell RNA sequencing (scRNA-seq)
This method is used to examine the transcriptome of individual cells and also known as Single cell transcriptome sequencing. It allows researchers to identify different cell types and subtypes based on gene expression patterns, as well as investigate gene regulation and cell signaling networks.
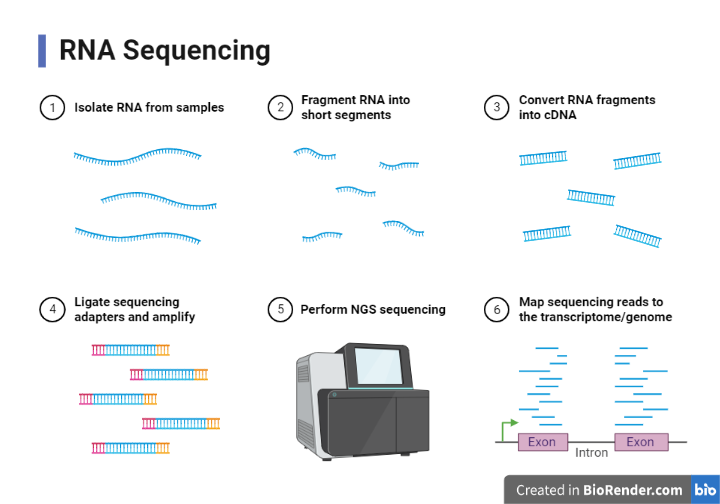
Fig: Workflow of RNA Sequencing
Single-cell DNA sequencing (scDNA-seq)
This method is used to sequence the genomes of individual cells. It allows researchers to discover genomic differences such as single nucleotide polymorphisms (SNPs), copy number variations (CNVs), and structural variants, as well as analyse clonal evolution and genetic heterogeneity.
Single-cell ATAC sequencing (scATAC-seq): This approach is used to examine the accessibility of chromatin in individual cells. It allows researchers to discover regulatory regions of the genome such as enhancers and promoters, as well as analyse gene regulation and epigenetic alterations.
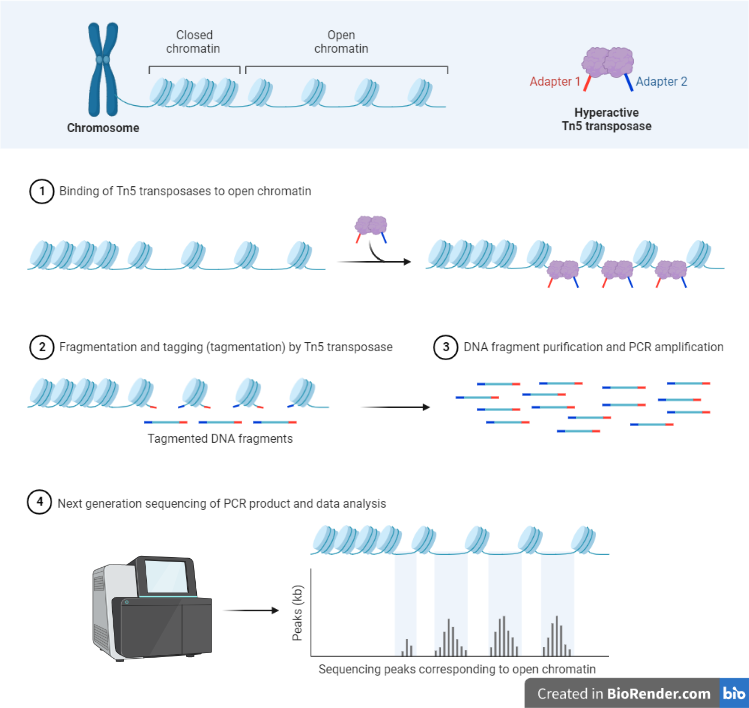
Fig: Workflow of ATAC Sequencing
Single-cell DNA methylome sequencing (scMethyl-seq)
This method is used to examine the methylation patterns of individual cells’ DNA. It enables scientists to investigate epigenetic changes and their impact in gene regulation, as well as to identify cell types and subtypes based on DNA methylation profiles.
Methods/Protocol:
Single-cell sequencing is a powerful approach for studying the genetic makeup of individual cells. The procedure begins with the isolation and amplification of DNA or RNA from a single cell, followed by high-throughput sequencing to acquire enormous quantities of sequence data.
Sample preparation: The first step in single-cell sequencing is to prepare the sample. This may involve obtaining tissue samples from a patient or animal model, or isolating individual cells from a culture or tissue. The cells are then washed to remove any debris or contaminants.
Cell capture: The next step is to capture individual cells for analysis. There are several methods available for this, including fluorescence-activated cell sorting (FACS), microdissection, or microfluidics. In general, the method used will depend on the sample type and the research question.
Cell lysis and RNA extraction: Once the cells are captured, they are lysed to release their contents, including RNA. RNA is extracted using a standard RNA extraction kit, which typically involves adding a lysis buffer to the cell lysate, followed by purification of the RNA using spin columns.
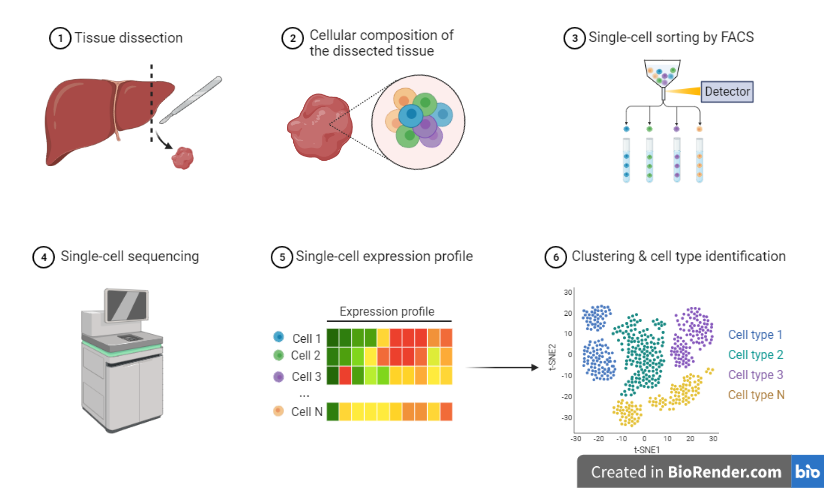
Fig: Workflow of Single Cell Genome Sequencing
Reverse transcription: The RNA is then converted into cDNA using reverse transcription. This involves adding reverse transcriptase and primers to the RNA sample to create complementary DNA strands.
Library preparation: The cDNA is then prepared for sequencing by creating a library. This involves fragmenting the cDNA, adding adapter sequences to the ends of the fragments, and PCR amplifying the library to increase the amount of material for sequencing.
Quality control: Before sequencing, the library is checked for quality and quantity. This may involve using a bioanalyzer or qPCR to assess the size distribution and concentration of the library.
Sequencing: The final step is to sequence the library using a high-throughput sequencing platform. The resulting sequence data can be analysed to identify gene expression patterns, mutations, and other genomic features of the individual cell.
Analysing single cell sequencing data:
Single-cell sequencing is an effective method for studying the genetic makeup of individual cells. Several steps are involved in analysing the resulting sequence data, including quality control, data normalization, clustering, differential expression analysis, and functional analysis. These stages are required to identify genes that are expressed differently in distinct cell kinds or groups, as well as biological pathways and processes that are enriched in different cell types or groups. While analysing single-cell sequencing data is a complex process that requires expertise in bioinformatics and computational biology, the insights gained can provide valuable information about the genetic makeup and function of individual cells, with applications in fields such as cancer research, developmental biology, and neuroscience.
Applications:
Single-cell sequencing has numerous applications in scientific domains such as cancer, developmental biology, neuroscience, immunology, personalized medicine, microbiology, and agriculture. The approach permits the identification of particular cells responsible for disease states, which can inform therapies as well as personalized medicines. It also provides insights into cellular variety, gene expression patterns, and functioning mechanisms of individual cells, allowing for a better understanding of complex biological systems.
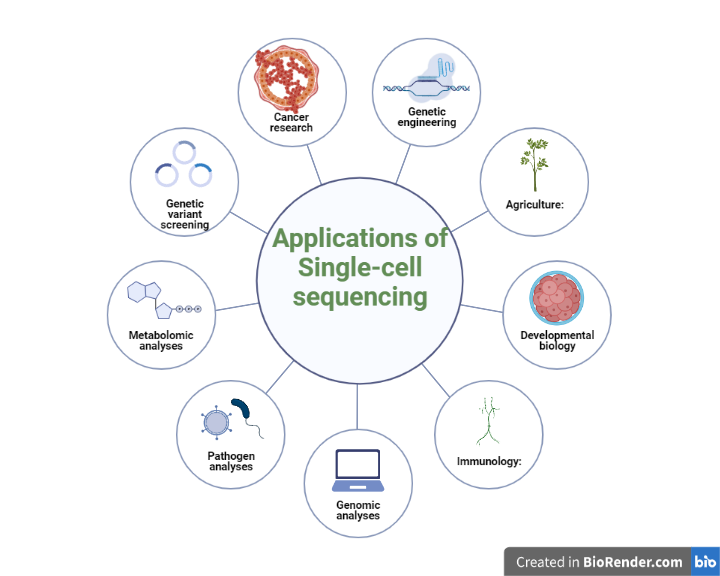
Fig: Applications of Single-cell sequencing
Limitations:
There are various limits to single-cell sequencing that researcher must consider when interpreting data and developing experiments. One of the most significant technical challenges is that single-cell sequencing is a complex procedure that necessitates specialized equipment, experience, and meticulous sample preparation. Furthermore, the high cost of single-cell sequencing can make it inaccessible and practical for some research projects. Another restriction is the low coverage of individual cells’ genomes, transcriptomes, or epigenomes, which can diminish data accuracy and precision. Data analysis can be difficult as well, especially when dealing with vast numbers of cells, distinct cell kinds, or complicated biological systems. Due to technical constraints, sample variance, or biological noise, there is also a possibility of false negatives and positives.
Finally, when cells are separated from their native tissue context during single-cell sequencing, spatial information is destroyed, making it difficult to study cell-to-cell interactions and tissue organization. Despite these limitations, single-cell sequencing remains an important tool for studying the genetic makeup and functional mechanisms of individual cells, and researchers are working to address these issues in order to improve the technique’s accuracy and reliability.
References:
- Jovic D, Liang X, Zeng H, Lin L, Xu F, Luo Y. Single-cell RNA sequencing technologies and applications: A brief overview. Clin Transl Med. 2022 Mar;12(3): e694.
- Tang, X., Huang, Y., Lei, J. et al. The single-cell sequencing: new developments and medical applications. Cell Biosci 9, 53 (2019).
- Haque, A., Engel, J., Teichmann, S.A. et al. A practical guide to single-cell RNA-sequencing for biomedical research and clinical applications. Genome Med 9, 75 (2017)
- Auerbach BJ, Hu J, Reilly MP, Li M. Applications of single-cell genomics and computational strategies to study common disease and population-level variation. Genome Res. 2021 Oct;31(10):1728-1741.